AI Tool Predicts Biological Age From Face Photos
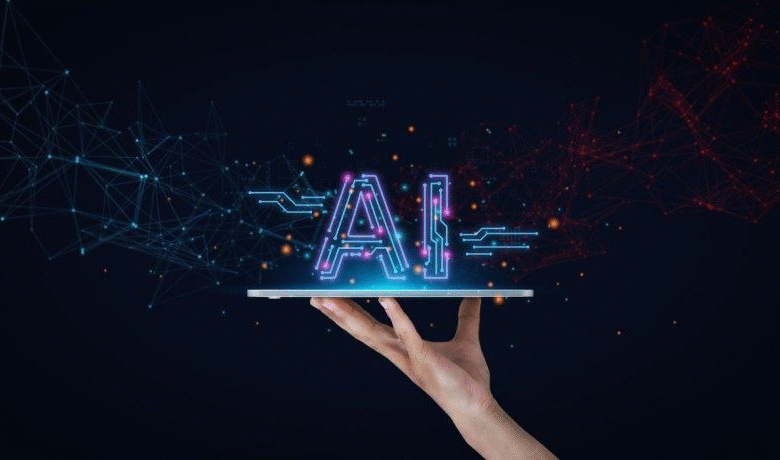
An innovative AI tool predicts biological age by analyzing facial photographs, offering a groundbreaking approach to health understanding and longevity. Developed by researchers at Mass General Brigham, this tool, known as FaceAge, utilizes advanced machine learning techniques to assess not just chronological age, but also the biological aging rate of individuals. By tapping into a database of over 58,000 images, FaceAge is capable of delivering health predictions that can influence clinical decisions, particularly in oncology and palliative care settings. The implications of such an aging analysis tool extend beyond mere curiosity; they promise to reshape how healthcare providers approach aging and treatment plans. As we navigate the complexities of biological versus chronological age, tools like FaceAge could revolutionize personalized medicine and enhance patient outcomes in the future.
The emergence of a new predictive technology in healthcare is promising to transform our understanding of aging. Utilizing deep learning algorithms, this advanced tool analyzes facial images to gauge health conditions and biological aging, providing crucial insights into a person’s wellness. As we delve into the realm of AI health predictions, these applications highlight a significant shift towards harnessing technology for medical advancements. By refining models that assess individual aging trajectories, this innovative solution may redefine the relationship between a person’s appearance and their overall health outcomes. As we explore how machine learning in healthcare can augment traditional practices, the distinction between biological and chronological age becomes increasingly vital to understanding our health.
Understanding FaceAge: A Revolutionary AI Tool for Biological Age Prediction
FaceAge is an innovative deep-learning algorithm developed by researchers at Mass General Brigham, designed to predict biological age from simple selfies. This AI health prediction tool takes into account the uniqueness of each individual, analyzing facial features to assess the rate at which a person is aging. Unlike chronological age, which is merely a number representing the years since birth, biological age can reflect an individual’s health condition and lifestyle choices, making it a more informative measure of one’s overall health.
The algorithm was trained using a vast dataset of 58,851 images, primarily from healthy individuals. This training allows FaceAge to provide insights that can potentially predict various health outcomes, including life expectancy and disease susceptibility. The implications of this AI-based aging analysis tool extend beyond mere curiosity; they could fundamentally change how healthcare providers assess patient health and tailor treatments, offering a more personalized approach to care.
The Impact of Biological vs Chronological Age in Healthcare
Understanding the difference between biological and chronological age is crucial in clinical settings. While chronological age simply marks the passage of time, biological age considers various factors such as genetics, lifestyle, and environmental influences that affect the aging process. This distinction is particularly vital for patients with chronic diseases, as biological age could provide insights into their resilience and recovery potential. Tools like FaceAge, which utilize machine learning in healthcare, can bridge this gap, offering healthcare professionals data-driven insights for decision-making.
By predicting biological age, FaceAge helps clinicians move beyond age-based biases that may influence their treatment plans. For example, an older cancer patient who appears younger biologically may respond better to certain therapies than younger patients with a higher biological age. Emphasizing biological over chronological age ensures that treatment decisions are based on individual health metrics rather than simplistic assumptions about age alone.
FaceAge: Transforming Outcomes for Cancer Patients
FaceAge’s accuracy in predicting biological age has significant implications for cancer care. In one study, the tool predicted that cancer patients had a biological age approximately five years older than their chronological age, which may influence treatment options and expected outcomes. This enhanced predictive power can aid oncologists in customizing treatment strategies that align with a patient’s overall health rather than relying solely on their birthdate.
Moreover, FaceAge outperformed clinicians in predicting survival outcomes for patients receiving palliative care, demonstrating its utility in managing complex health situations. By providing a more precise understanding of a patient’s biological condition, healthcare providers can prioritize interventions that focus on improving quality of life, potentially transforming the future landscape of oncology.
Machine Learning and Its Role in Predicting Health Outcomes
Machine learning in healthcare is at the frontier of transforming how medical professionals approach disease prediction and management. Platforms like FaceAge leverage vast data sets to create algorithms capable of analyzing images with remarkable precision. This deep-learning capability does not only offer biological age predictions but also raises the potential for broader applications, including detecting early signs of diseases and assessing general health status.
Healthcare systems are increasingly adopting such technologies to enhance patient care. As predictive models become more sophisticated, tools like FaceAge can play a role in preventative medicine, allowing physicians to implement proactive strategies that address health concerns before they escalate. This shift towards early detection and personalized treatment highlights the growing significance of machine learning in fostering better health outcomes.
Ethical Considerations Surrounding AI Health Predictions
As promising as AI tools like FaceAge are, they also raise important ethical considerations. Questions about data privacy, consent, and ownership of facial data are evolving alongside these technological advancements. For instance, patients must be informed about how their images will be used and analyzed. Ensuring that individuals understand the implications of their facial data being processed is essential for fostering trust in these AI predictions.
Another point of concern is the potential for bias in AI-generated outcomes. If the training data lacks diversity, the risk of skewed results increases, which could disproportionately affect certain populations. It is crucial for developers to create algorithms that offer equitable health predictions, as relying solely on AI could unintentionally perpetuate existing disparities in healthcare.
The Psychological Impacts of Predictive Aging Tools
The introduction of AI tools like FaceAge may also have unforeseen psychological impacts on individuals. For instance, being told that one appears older than their chronological age could alter self-perception and potentially influence mental health. The concept of biological age, when communicated without context, might invoke anxiety or concern among patients regarding their health status and longevity.
It becomes vital for healthcare professionals to provide adequate context when discussing AI predictions. Patients deserve transparency about how these results may affect their treatment options and overall health perceptions. By ensuring that the implications of biological age assessments are properly framed, clinicians can help mitigate the psychological effects that may arise from these advanced technologies.
Future Directions for AI in Aging and Health Prediction
Looking ahead, the integration of AI tools like FaceAge in clinical settings heralds a new era of healthcare that prioritizes precision medicine. Future research endeavors will likely involve expanding the diversity of patient data to enhance the model’s predictive capabilities and reduce biases. As ongoing studies evaluate the effectiveness of FaceAge across various demographics and health conditions, its potential applications may vary widely, offering insights far beyond aging predictions.
Moreover, the increasing acceptance of AI solutions in healthcare might lead to the development of additional AI health prediction tools that can address various aspects of patient care, including chronic disease management, lifestyle adjustments, and even early detection of mental health issues. The proactive utilization of these technologies could redefine patient engagement strategies, making it possible to foster healthier populations through informed decision-making.
Collaboration Between AI and Human Judgment in Healthcare
While AI tools like FaceAge show tremendous promise, experts emphasize that they should complement, not replace, human judgment. The integration of machine learning into healthcare allows clinicians to leverage data that supports clinical instincts rather than substituting them entirely. This collaborative approach ensures that a patient’s dignity and personalized care remain central to medical decisions.
Moreover, as Dr. Harvey Castro pointed out, even with advanced AI, the essence of healthcare lies in empathy, context, and humanity. It is essential for healthcare professionals to remain at the forefront of decision-making, using AI as an invaluable resource that enhances their ability to care for patients rather than diminishing it. This balance between technology and human insight will be critical as the healthcare landscape continues to evolve.
Leveraging AI for Early Detection and Prevention
The predictive capabilities of AI tools like FaceAge could revolutionize early detection strategies in medicine. The ability to assess biological age through facial analysis not only accelerates diagnosis but also enhances preventive measures. By identifying aging trends or health risks efficiently, healthcare providers can initiate timely interventions that might curb the progression of diseases.
As technologies develop, integrating AI into routine health screenings could become an invaluable practice, allowing providers to monitor patients’ aging processes over time. Simple selfie analyses might eventually transform how preventive healthcare is practiced, encouraging a shift toward proactive health management that prioritizes individual wellness before concerns arise.
Frequently Asked Questions
What is the FaceAge AI tool and how does it predict biological age?
The FaceAge AI tool is an advanced aging analysis tool developed by researchers at Mass General Brigham that uses deep learning algorithms to analyze facial photos. By evaluating features in a selfie, FaceAge predicts an individual’s biological age, which reflects their actual aging process as opposed to their chronological age. This tool can provide insights into health outcomes and life expectancy.
How accurate is the FaceAge AI tool compared to human predictions in healthcare?
In studies, the FaceAge AI tool demonstrated a higher accuracy in predicting biological age and survival outcomes compared to clinicians’ predictions. It successfully estimated biological ages that were about five years older than patients’ chronological ages, particularly among cancer patients, showcasing its potential as a reliable AI health prediction tool.
Can FaceAge be used in clinical settings for decision making?
Yes, FaceAge is designed to support clinical decision-making by providing objective biological age assessments based on facial analysis. While it can enhance predictions about survival and personalize care plans, researchers emphasize that it should augment rather than replace human judgment in healthcare.
What training data was used for the FaceAge AI tool?
FaceAge was trained using a dataset of 58,851 photos collected from presumed healthy individuals. This diverse training data enables the AI tool to learn and predict biological age accurately, but concerns about data diversity and potential biases remain.
What potential ethical considerations arise from using the FaceAge AI tool?
The application of the FaceAge AI tool raises various ethical questions, including concerns about data privacy, consent regarding facial data usage, and the implications of being told one ‘looks older’ than their chronological age. Ensuring that this technology is used within a strong regulatory and ethical framework is crucial for its success in healthcare.
How might the FaceAge tool impact palliative care and treatment decisions?
FaceAge has the potential to improve palliative care in oncology by helping to prioritize treatment based on a patient’s biological age rather than chronological age. This personalization can lead to more effective care, especially in patients whose resilience is paramount for their treatment outcomes.
What are the future research directions for the FaceAge AI tool?
Future research on FaceAge aims to explore its predictive capabilities for various chronic diseases, general health status, and lifespan across different populations and health conditions, particularly in cancer patients at various stages. This work will help evaluate its broader applicability and effectiveness as an aging analysis tool.
How does FaceAge differ from traditional age assessment methods?
Unlike traditional age assessments that rely on medical history and clinical evaluations, FaceAge uses facial features obtained from a simple photo to predict biological age. This innovative approach combines machine learning in healthcare with advanced imaging analysis, making the assessment process more objective and accessible.
What is the significance of distinguishing biological age from chronological age?
Distinguishing between biological age and chronological age is significant as it provides a deeper understanding of an individual’s health trajectory. Biological age reflects the body’s physiological state and potential health risks, allowing for more tailored healthcare interventions and lifestyle recommendations, particularly for aging and chronic disease management.
Can anyone use the FaceAge tool, or is it restricted to certain populations?
Currently, the FaceAge tool’s application may be focused on certain populations, such as cancer patients, as part of ongoing research. However, the hope is that with further development and validation, it could eventually be accessible to a broader audience as a preventive health tool for aging analysis.
Key Points | Details |
---|---|
AI Tool: FaceAge | A deep-learning algorithm that predicts biological age from facial photos. |
Functionality | Analyzes selfies to estimate aging rates and predict health outcomes, especially for cancer patients. |
Studies Conducted | Trained on 58,851 photos; accuracy tested on 6,196 cancer patients and 100 palliative care patients. |
Clinical Application | Aims to complement clinician judgments, aiding in personalized treatment planning. |
Potential Concerns | Data bias, ethical issues regarding data ownership and patient consent. |
Future Research | Will involve diverse patient populations to enhance predictive capabilities. |
Summary
The AI tool that predicts biological age is a groundbreaking innovation that analyzes facial photographs to assess an individual’s health outcomes and aging rate. Developed by Mass General Brigham, FaceAge utilizes deep-learning algorithms to offer predictions that can significantly impact clinical decision-making in healthcare. This technology not only forecasts biological age but also holds promise for improving treatment plans, particularly in oncology. As research continues to address ethical and data diversity concerns, the potential applications of FaceAge may extend well beyond mere age estimation, paving the way for enhanced patient care.